Ruiqi Gao
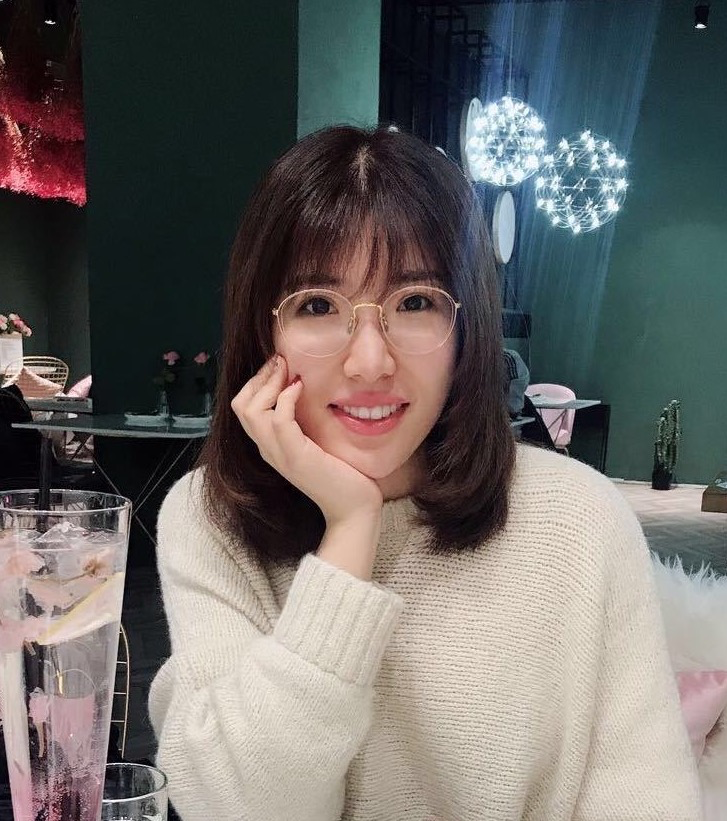
I am a Research Scientist at Google DeepMind. I am mainly interested in generative models and representation learning. Specifically, I work on efficient and scalable learning algorithms of deep generative models, such as diffusion models and energy-based models. I also work on representational models with implications in neuroscience. I obtained my Ph.D. from UCLA advised by Song-Chun Zhu and Ying Nian Wu. Prior to that, I received my B.S. degree of Statistics from Peking University.
Contact: ruiqimgao at gmail dot com
Highlight research themes:
(1) Maximum likelihood learning of deep generative models.
- Diffusion recovery likelihood of EBMs (Gao et al., ICLR 2021).
- Multi-grid learning of EBMs (Gao et al., CVPR 2018).
- Spatial-temporal top-down models (Xie*, Gao*, et al., AAAI 2020), (Xie*, Gao*, et al., AAAI 2019).
(2) Joint learning of various models.
- Contrastive learning of EBMs with flow-based models (Gao et al., CVPR 2020).
- Mixing MCMC of EBMs with flow-based models as backbones (Nijkamp*, Gao*, et al., arXiv 2020).
- Cooperative learning of EBMs with top-down models (Xie et al., AAAI 2019 & TPAMI).
(3) Representational models with implications in neuroscience.
- Representational models of grid cells (Gao et al., NeurIPS 2021), (Gao et al., ICLR 2019) and applications in 3D vision (Zhu et al., CVPR 2021).
- Representational models of V1 cells and applications in motion inference (Gao et al., AAAI 2022).